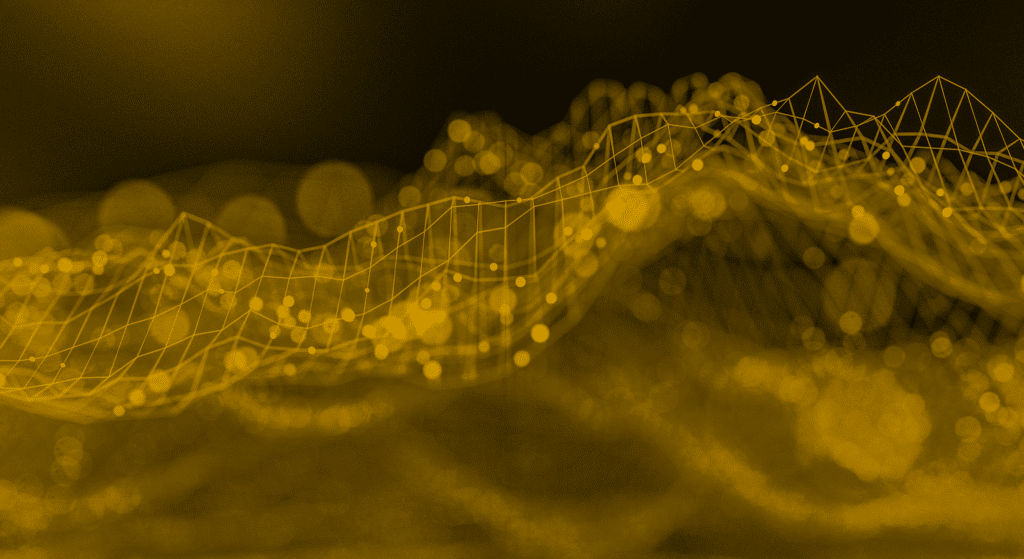
High-end Edge AI Requirements
February 22, 2021
Challenges of the High-End Edge AI Market
At Mythic, we have pioneered analog compute for high-end edge AI applications. To answer the question “why analog compute?”, it is best to first understand the challenges Mythic is solving for our customers. Our customers are trying to solve immense technical challenges that take in huge amounts of sensor data and then compute on the order of many trillion of operations per second. These customers are also constrained by economics and physics of a volume Edge AI product deployed out in the world. Let us break down the six factors that our customers care about, with three of them focused on performance and three focused on constraints at the edge.
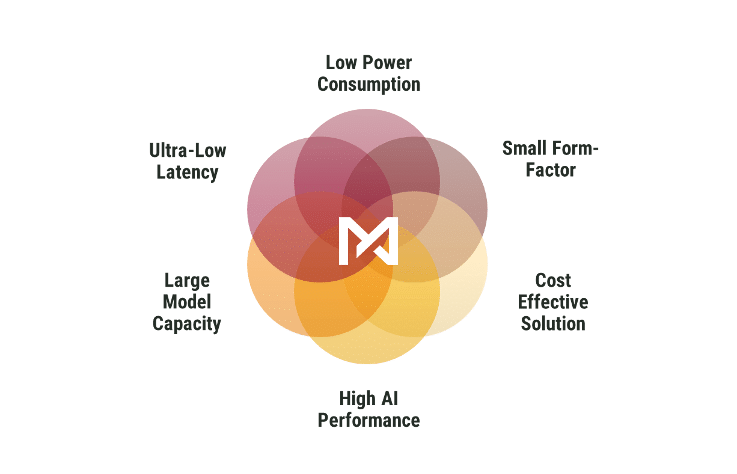
Three High-Performance Requirements for Edge AI
Frame Rate, Latency, and Resolution
The opportunity to deliver high-quality AI at the edge starts with the performance of the AI accelerator. Operating at high frame rates (60-120 fps) and high resolutions (HD to 4K) provides significantly better inference results. Low latencies (<10ms) are critical for immersive AR/VR experiences, faster decision-making, and the ability for smart cameras to enhance the image quality
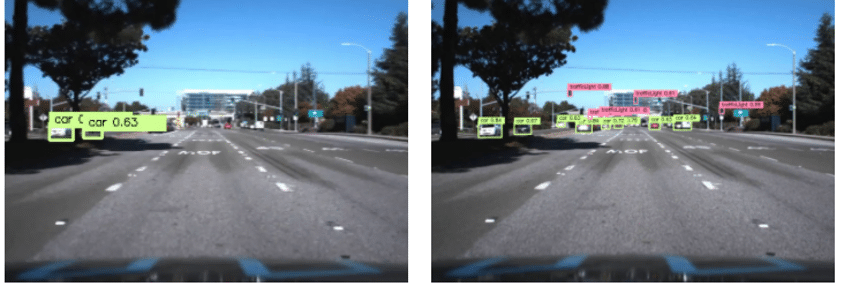
State-of-the-Art Models
State-of-the-art in AI has moved quickly over the years. Models like VGG and ResNet have been surpassed by newer models like EfficientNet and FBNet. Object detection models like FasterRCNN have been surpassed by YoloV3, then YoloV4, and now EfficientDet. Powerful transformer models with hundreds of millions and billions of weights that power search, recommendation, and NLP in the datacenter will ultimately find their way into edge devices.
High-end edge AI accelerators must be future-proof to this evolution. On top of needing a massive amount of weight storage and raw matrix multiplication horsepower, the AI accelerator and its compiler must also be flexible enough to support changing compute paradigms in AI.
Deterministic Execution and Concurrency
Concurrency takes performance challenges to the next level. While the benchmarking world in AI fixates on single models running peak performance, the real world is far more complicated. Customers want to design for a guaranteed latency at compile time. They want to deterministically run three to five models in parallel without losing the <10ms latency of each model. If the workload suddenly increases (say a dozen cars drive past a license plate reader), the system should not skip a beat. As the complexity and capabilities of AI increase in the coming years, this challenge will only get more difficult.
Three Real-world Constraints for Edge AI
Low Power
Top of mind for all edge product designers is power. For battery-powered devices, power consumption is critical since it determines how long a device can run and what it can do. Even for devices with wall power, though, there are tight power constraints. Most edge devices are compact, with tight heat dissipation requirements. They may be powered with Power-over-Ethernet (PoE), which has limited power budgets. Power is not a simple concept either. A lot of benchmark attention is on power draw during peak performance (typically called TOPS/W, or Inferences/W), but in the real world what matters more is power scalability. Strong performance is needed at 0.5W, 2W, or whatever the system can allocate. When nothing is happening, power should be as close to zero as possible, and it should be quick and easy to switch between these different modes.
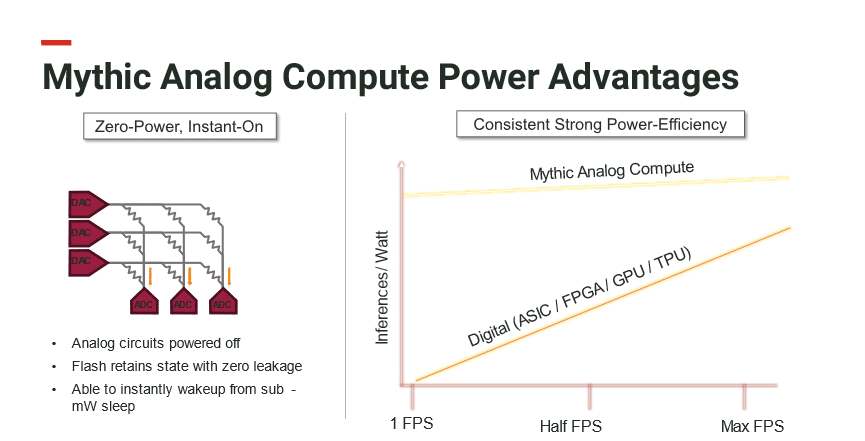
Small Size
Some customers prefer to run AI algorithms at the far edge of the network, at the source of the data. Here, the latency is minimized, and there is no loss of accuracy due to video compression. In these edge systems, there is no room for large PCIe cards, big heatsinks, or fans. In some systems, the entire inference engine needs to fit on a 22mm x 30mm M.2 A+E card or a half-height mini-PCIe card that slots into a very crowded motherboard. Even with edge-servers that accept larger PCIe cards, the size of the accelerator and cooling solution dictates how much AI compute can be packed in.
Cost-effective
Edge AI accelerators must deliver high-end performance at a cost-effective price point. AI accelerator prices must be such that they fit into systems that retail starting from $300. For edge-servers, processor or card price is less important, but solutions that offer > 10X compute per dollar advantages against GPUs gives end customers much greater ability to scale to customer demand.
Analog in a Digital AI World
Across the spectrum of AI accelerator options, all-digital solutions consistently fall short. Either the solutions are too expensive, or the performance is too low to meet customer needs. To deliver an effective AI accelerator solution that satisfies on power, performance, and cost, truly unique technology is needed.
In the digital world, even with clever architectures, memory is the main bottleneck. Squeezing more performance in the presence of severe memory bottlenecks quickly drives up the power and system cost, making it incredibly difficult to address all six Edge AI factors. At Mythic, we use analog compute to perform computations inside incredibly dense flash memory arrays. The performance, power, and cost advantages make our solution incredibly unique in a crowded market space.
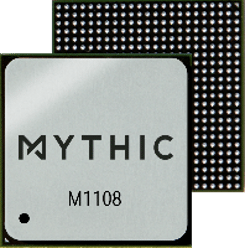
To learn more about analog compute, check out this blog post from our CTO: https://mythic.ai/mythic-hot-chips-2018/.
If you want to see our analog compute solution in action, including the huge technical hurdles we had to overcome, check out this blog post: https://mythic.ai/the-era-of-analog-compute-has-arrived/
Want to hear from Mythic?
Get the latest updates on news, events and blog post notifications! Subscribe to our What’s New Newsletter.
You can unsubscribe at any time by clicking the link in the footer of our emails. For information about our privacy practices, please view our privacy policy here.